일 | 월 | 화 | 수 | 목 | 금 | 토 |
---|---|---|---|---|---|---|
1 | 2 | 3 | 4 | 5 | ||
6 | 7 | 8 | 9 | 10 | 11 | 12 |
13 | 14 | 15 | 16 | 17 | 18 | 19 |
20 | 21 | 22 | 23 | 24 | 25 | 26 |
27 | 28 | 29 | 30 |
- center loss
- 큐
- Knowledge Distillation
- 딥러닝
- 논문 구현
- Object Detection
- Computer Vision
- flame
- Object Tracking
- deep learning
- level2
- reconstruction
- 스택
- 프로그래머스
- attention
- 자료구조
- Deeplearning
- OpenCV
- re-identification
- Threshold
- 3D
- 알고리즘
- 임계처리
- Python
- NLP
- transformer
- 파이썬
- numpy
- cv2
- point cloud
- Today
- Total
목록딥러닝/3D (4)
공돌이 공룡의 서재
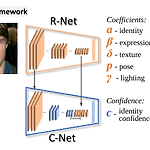
Introduction 사용할 수 있는 3D Face data 가 많지 않다는 것이 문제다. 그래서 이전 연구들은 surrogate shape label 과 같은 고전적인 방법들로 맞춰진 3D shape 이나 합성 데이터를 사용하는 방식을 택했다. 이 방식의 문제는 Domain gap 이 남아있다는 점이랑 label 이 완벽하지 않다는 점이다. 이런 이슈를 해결하기 위해서 라벨 없이 unsupervised 또는 weakly supervised 방식을 사용하는 연구들이 많이 나왔다. 이 연구들의 핵심은 미분 가능한 이미지를 형성하는 것인데, 얼굴 이미지를 네트워크의 예측 결과를 이용해서 렌더링하기 때문에 가능하다. 또한 입력 이미지와 렌더링된 이미지의 차이를 supervision (weakly 인듯) 으로..
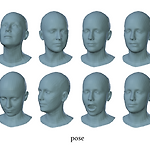
3D Face Reconstruction 에서 최근에 등장하여 3DMM 모델과 함께 많이 쓰이고 있다. 사실 FLAME 을 이용해서 어떻게 Reconstruction 을 할지가 더 중요하긴한데, 그래도 한번 살펴보자. 💡 FLAME = Faces Learned with an Articulated Model and Expressions : use 33000 scans : combines linear shape space with an articulated jaw, neck, and eyeballs, pose-dependent corrective blendshapes, and additional global expression blendshapes : low-dimensional but more expres..
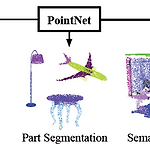
PointNet: Deep Learning on Point Sets for 3D Classification and Segmentation https://arxiv.org/abs/1612.00593 글을 쓰는 시점에서 인용수가 5천이 넘었다. input으로 point cloud를 직접적으로 다룬 딥러닝 모델이다. 딥러닝 모델에서 point cloud를 다루기 위해서 무엇이 필요한지를 잘 설명하고 있다. Introduction point cloud는 정해진 format이 없기 때문에, 전에 있던 대부분의 연구가 이를 3D voxel grid로 바꾸거나 image들의 집합으로 바꾸고 난 후에 딥러닝 모델에 넣었다. 그러나 이렇게 처리하는 것은 데이터의 natural invariance를 가릴 수 있는 quan..
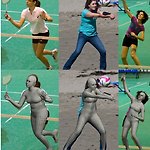
Expressive Body Capture: 3D Hands, Face, and Body from a Single Image https://arxiv.org/abs/1904.05866?utm_source=feedburner&utm_medium=feed&utm_campaign=Feed%3A+arxiv%2FQSXk+%28ExcitingAds%21+cs+updates+on+arXiv.org%29 [1] Introduction 사람의 행동을 더 잘 이해하기 위해선, 사람의 major keypoint들을 estimation 하는 것으로는 충분하지 않고, 몸, 손, 얼굴의 3D surface 정보들까지 포함해야 할 필요가 있다. 그런데 적절한 3D model과 3D training data가 충분하지 않아서, 이렇..